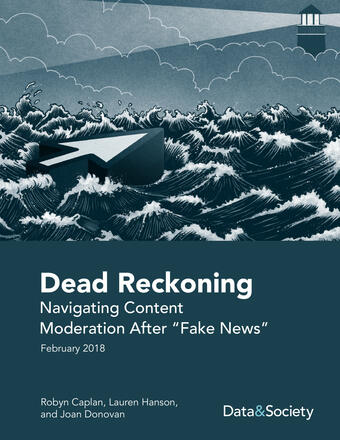
Related contents
Fake news. It’s complicated.A multi-dimensional approach to disinformation
Information disorder: Toward an interdisciplinary framework for research and policy making
Information Disorder: The Essential Glossary
The term “fake news” is everywhere, but its meaning is contested. It has two main uses: “fake news” as critique of “mainstream media” (a term which has itself been used as a critique of the media of the “liberal elites” for more time than “fake news”), that are accused of being biased against the right; “fake news” as problematic content using news signifiers.
Various attempts have been made to use more precise terminology. One approach makes distinctions based on the intention of the creator, but it is not always possible to know the intent, and thus to distinguish deliberate deception from satire or from a simple mistake. Other approaches include factors such as strategy and style of presentation. Drawing clear lines remains difficult, e.g. satire disclaimers may exist, but only to prevent litigation, or they may have been added a long time after the creation of a website.
Some recent approaches are feature based, focusing on making it possible for human moderators or machine learning systems to detect “fake news”. Those systems require significant investments, which are possible only for platforms like Facebook and Google. There are many problems. Platforms don’t share much information about the mechanisms they use. Universal standards may result in false positives. Indicators of trustworthiness may marginalise mid-level blogs or websites. Established media organisations sometimes use the same viral marketing techniques as fake news websites.
There are four emerging strategies for intervention. Trust and verification/fact-checking: the approach is based on the assumption that determining whether something is true or false requires experts and professionals. Trust marks, such as Twitter’s blue check, may be used. Demonetization disrupts economic incentives to create fake news, but it is unclear how Facebook and Google are implementing their policy. Moreover, fake news and hyper-partisan websites are able to modify their strategy to keep making money. De-prioritizating content and banning accounts, using humans or algorithms, leaves discretion to those who make the policy, write the algorithms, or make the decisions. Supporters of regulatory approaches believe that platforms' intervention is not enough and governments should address “fake news” and hate speech online. In June 2017, Germany passed the NetzDG law, requiring social media platforms to remove hate speech and criminal material within 24 hours after receiving a notification or complaint, or to block the offending content within seven days. Social media companies that persistently fail to remove illegal content face fines of up to 50 million Euros.
Tags: Fake news and disinformation Germany Fact-checking CensorshipThe content of this article can be used according to the terms of Creative Commons: Attribution-NonCommercial 4.0 International (CC BY-NC 4.0) . To do so use the the wording "this article was originally published on the Resource Centre on Media Freedom in Europe" including a direct active link to the original article page.