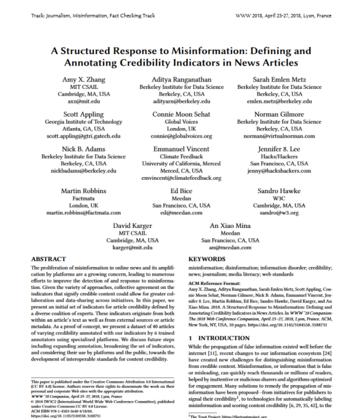
The authors describe a set of initial indicators for article credibility, grouped into content signals (determined by considering only the text or content of an article), and context signals (determined through consulting external sources or article metadata) which were iteratively developed through consultation with different stakeholders and require human judgement and training. The focus of the work is therefore based on broadening participation in credibility annotation and improving media literacy.
To validate the indicators and examine how they get annotated, researchers gathered a dataset of 40 highly shared articles focused on two topics possessing a high degree of misinformation in popular media: public health and climate science. Each of these articles was annotated with credibility indicators by 6 annotators with training in journalism and logic and reasoning, but with no domain expertise. Indicators related to publishers, authors, or any multimedia content were ignored.
From a collection of 12 major categories - including reader behavior, revenue models, publication metadata, and inbound and outbound references - 16 indicators were chosen for annotation, divided in 8 content and in 8 context indicators.
Content indicators, defined as those that can be determined by analysing the title and text of the article without consulting outside sources or metadata, included:
- Title Representativeness
- “Clickbait” Title
- Quotes from Outside Experts
- Citation of Organisations and Studies
- Calibration of Confidence
- Logical Fallacies
- Tone
- Inference
Context indicators, on the other hand, require annotators to look outside the article text and research external sources or examine the metadata surrounding the article text, such as advertising and layout. These included:
- Originality
- Fact-checked
- Representative Citations
- Reputation of Citations
- Number of Ads
- Number of Social Calls
- “Spammy” Ads
- Placement of Ads and Social Calls
The study was also conducted by deploying several online tools or software platforms such as BuzzSumo (to choose the articles with higher impact); Check (to support the annotation of content indicators); Archive.is (to save the selected articles).
The research offers a first example of how collectively agreed indicators and data-sharing across initiatives could be used to build models of credibility that are both interpretable and robust to manipulation. It also states that the work aims at expanding its range, also seeking to shrink gaps of understanding between domain expertise and public knowledge.
Finally, it acknowledges that even in case of success in curbing some of the psychological foundations of misinformation, such as frequency of exposure, more work is needed to fully address the many social and identity-related motivations for believing misinformation.
Tags: Fake news and disinformation Media literacy Ethics of journalismThe content of this article can be used according to the terms of Creative Commons: Attribution-NonCommercial 4.0 International (CC BY-NC 4.0) . To do so use the the wording "this article was originally published on the Resource Centre on Media Freedom in Europe" including a direct active link to the original article page.